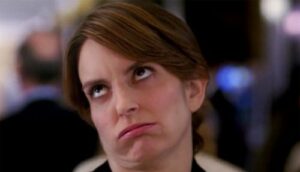
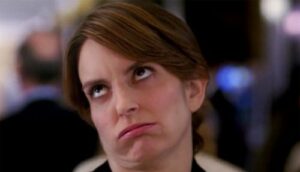
You Think You Have Clean Data. Commence Eye Roll.
Back in Q1, we started a program to help a client drive webinar attendees. The goal was to host a weekly webinar lead by the CEO that would have a handful of highly qualified attendees, instead of the traditional large webinar strategy. Metrics showed their past webinar attendees had strong conversion to closed-won sales. They just needed more.
As we started the program, the client felt confident in the quality of the lists they had sourced from data.com.
Sigh. We’ve heard that many times before.
We started calling based on the data and noticed several issues. First, the conversion from leads attempted to webinar attendees was less than 3 percent. Second, the invalid rate on the list was 34 percent, which meant at least three out of 10 of the first call attempts were completely wasted. Finally, we had high referral rates coming out of our conversations, which meant we had to spend a lot of time navigating to the correct person.
We convinced the client to spend part of their investment to cleanse the data to see if we could improve the conversion rate. We not only made sure the contact information like email and phone were accurate, we also captured the exact relevant title of focus.
The results were dramatic. With the newly cleansed data, the response rates more than doubled to 7.2 percent. Even though the client spent almost $3,000 to cleanse the list, the overall cost per lead (CPL) dropped by nearly $200 per attendee. (See chart below)
CPL was lowered by 26 percent by spending $3,000 to clean the prospect list.
It’s valuable to invest in data sources like data.com, SalesLoft, Hoovers, DiscoverOrg, etc. Those sources are best used as a starting source that will be enhanced to be more accurate and relevant for the needs of the campaign. Let’s be honest: no one likes to spend more money than they need to on data. However, if a prospecting team is spending time navigating and calling the wrong people, you will be surprised by the negative impact that has on CPL. Use metrics to justify the investment in data cleansing, making sure to account for wasted time and opportunity cost.